Understanding Different Types of Risk Intelligence Data
From sanctions to more sophisticated AI-driven solutions. An outline of the nuances of each risk data category and its relevance for compliance practices.
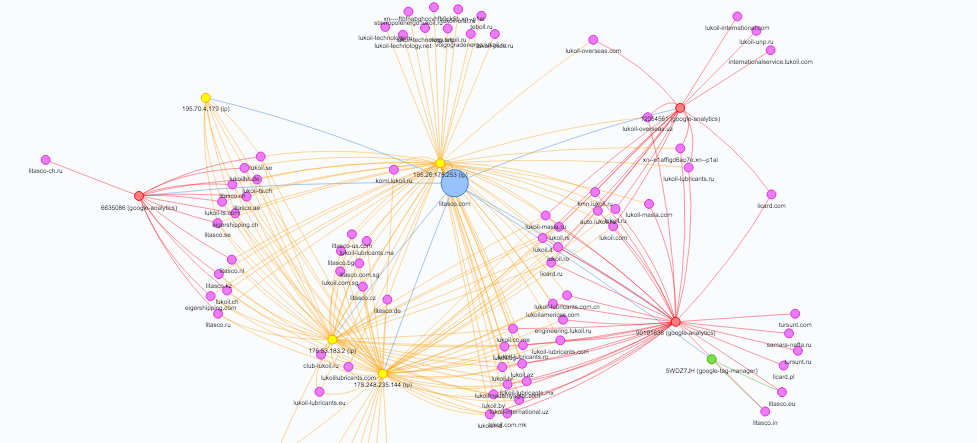
It has been almost 25 years since the first Risk Intelligence Databases entered the market with the goal of allowing financial institutions and corporations to identify customer and third-party risk. Since that time the Internet and the access to information has increased by many orders of magnitude, and has led to a landscape of different risk data offerings. These different categories of risk data serve distinct purposes, ranging from basic sanction screenings to more sophisticated AI-driven solutions.
The below outlines the nuances of each risk data category and its relevance for compliance practices.
Sanctions & Watchlist Data
This category encompasses structured information sourced primarily from government and open-source sanction and watchlists. While the data itself may seem standardized, providers enhance its value through advanced name matching techniques, minimizing false positives, and ensuring continuous updates and standardization of underlying sources.
Use Case: Sanctions and watchlist data screening forms the foundational level of compliance data, serving as a crucial requirement for companies to adhere to sanction regulations.
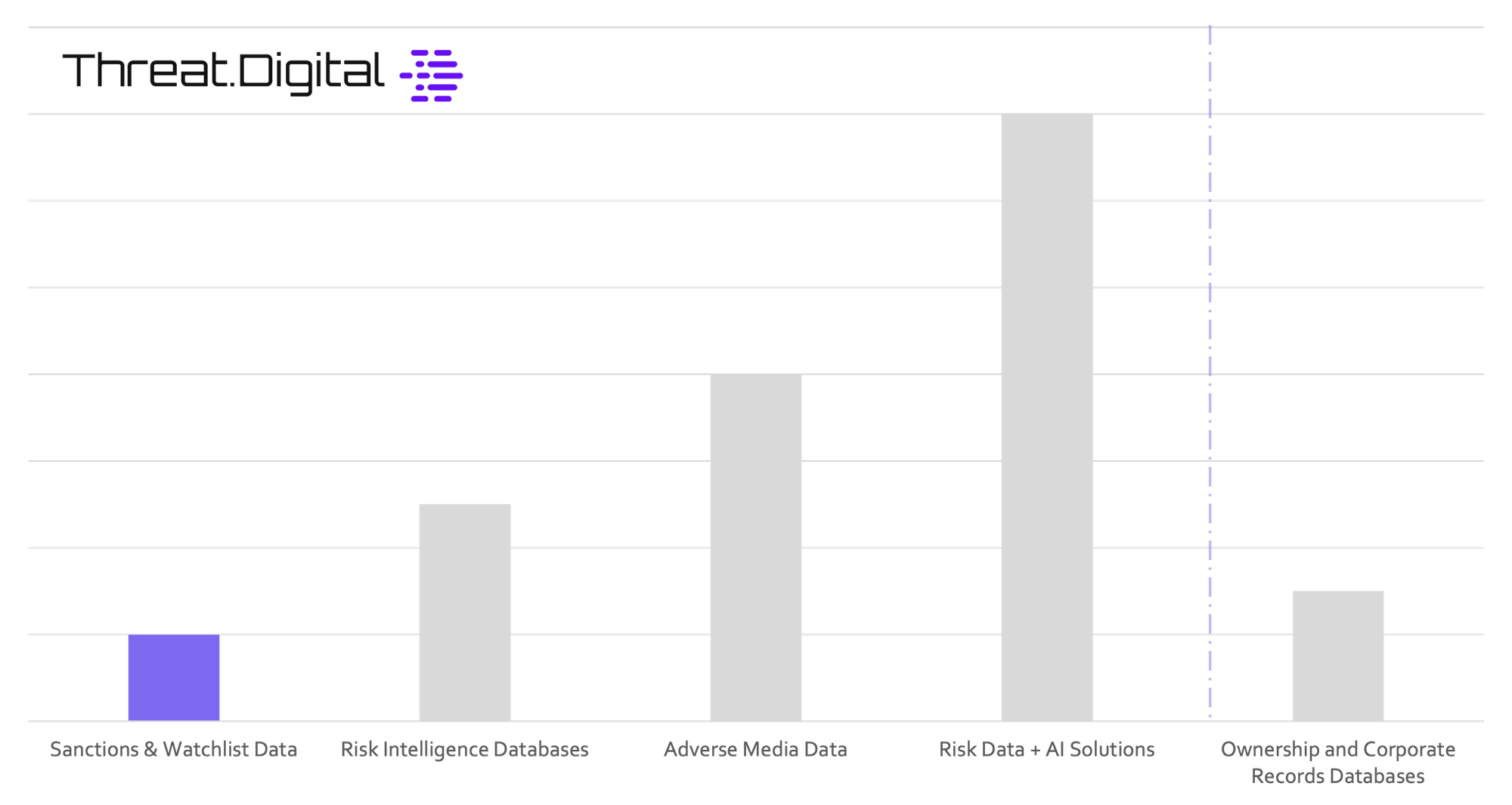
Risk Intelligence Databases
Human-curated structured profiles of entities and individuals categorized by risk levels constitute this data type. Besides sanctions, these databases include other risk categories such as politically exposed persons (PEPs), limited adverse media, and in some cases state-owned enterprises (SOEs). Profiles are consolidated, offering a comprehensive view. Providers typically offer between 1 to 10 million profiles.
Use Case: Offering a step up from sanction screening, these databases provide more in-depth risk information, covering PEPs and some adverse media events.
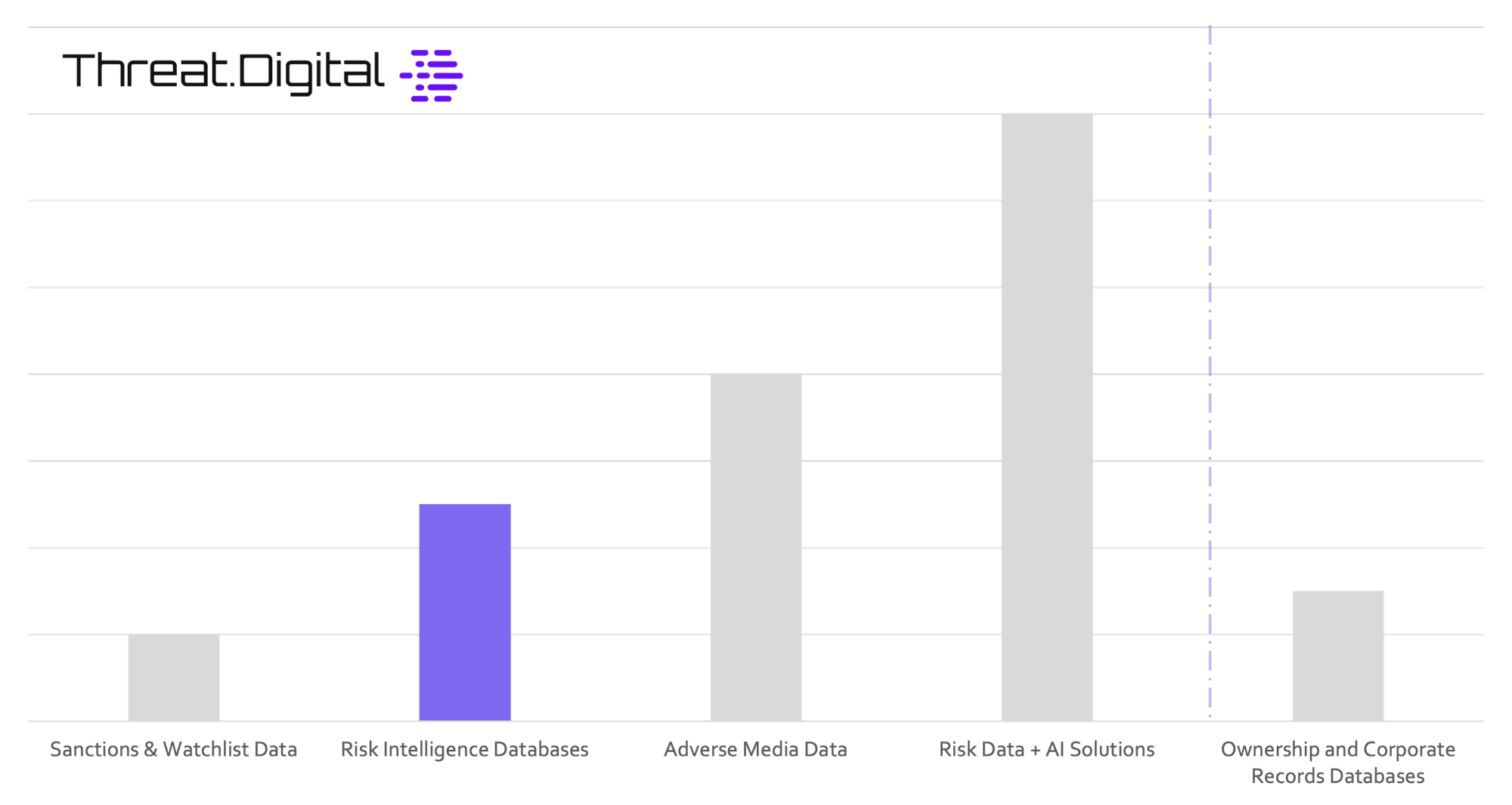
Adverse Media Data
Adverse media data generally refers to unstructured news or web content focusing on risk events. These databases are leveraged because they contain significantly more information than what is available in Risk Intelligence Databases, which will also have some limited amount of adverse media events. Although the coverage of information is greater in these datasets, the volume of information (and unstructured format) make them difficult to use for larger scale screening where false positives are a concern.
Use Case: Primarily utilized for ad hoc searches or complementing structured data sources, adverse media data helps uncover information missed elsewhere and enhances risk coverage.
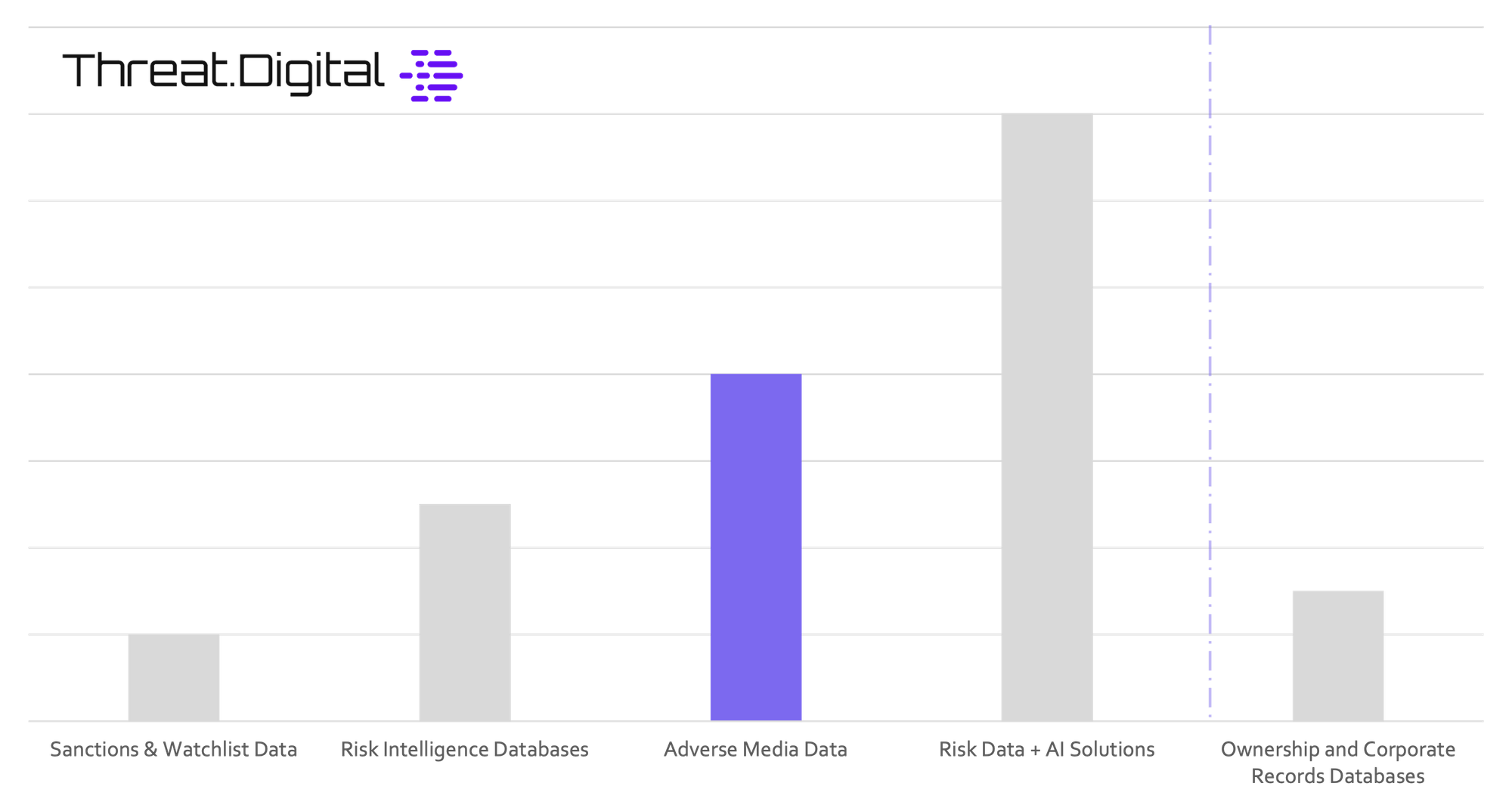
Risk Data + AI Solutions
This category amalgamates many of the traditional risk data sources outlined above, usually with a higher focus on news and unstructured information, and combines machine learning (e.g. AI) to improve coverage and even reduce false positives. Many of the solution providers in this category are newer startups that use AI applied to a high degree, and in some cases even leverage large language models. These tools are the first step towards having technology identify risk events previously only found in human level research.
Use Case: Acting either as a standalone solution or supplementing existing data, these tools offer broader coverage and scalability while mitigating false positives. They can also aid in cost reduction by replacing lower level due diligence reports/research.
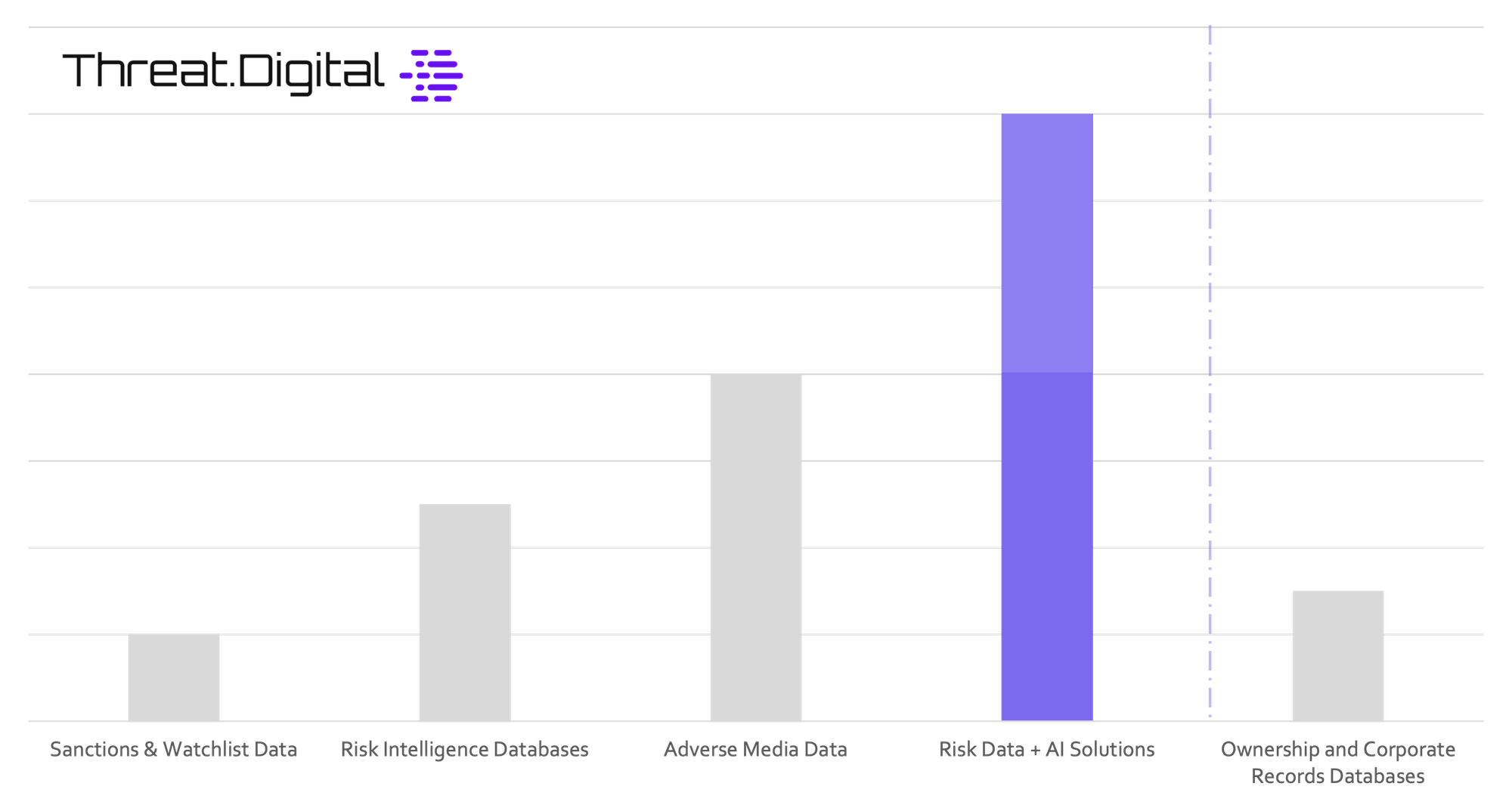
Ownership and Corporate Records Databases
Data in this category includes aggregate global corporate records to identity company ownership. In some cases, the providers of this data may also supplement these datasets with expert research, or obtain self reported company details. These data sources don't have broad risk application like many of the above solutions, but they serve to help to comply with specific and important sets of regulations.
Use Case: Crucial for compliance with regulations related to company ownership identification, such as OFAC sanctions rules or understanding supply chain risks.
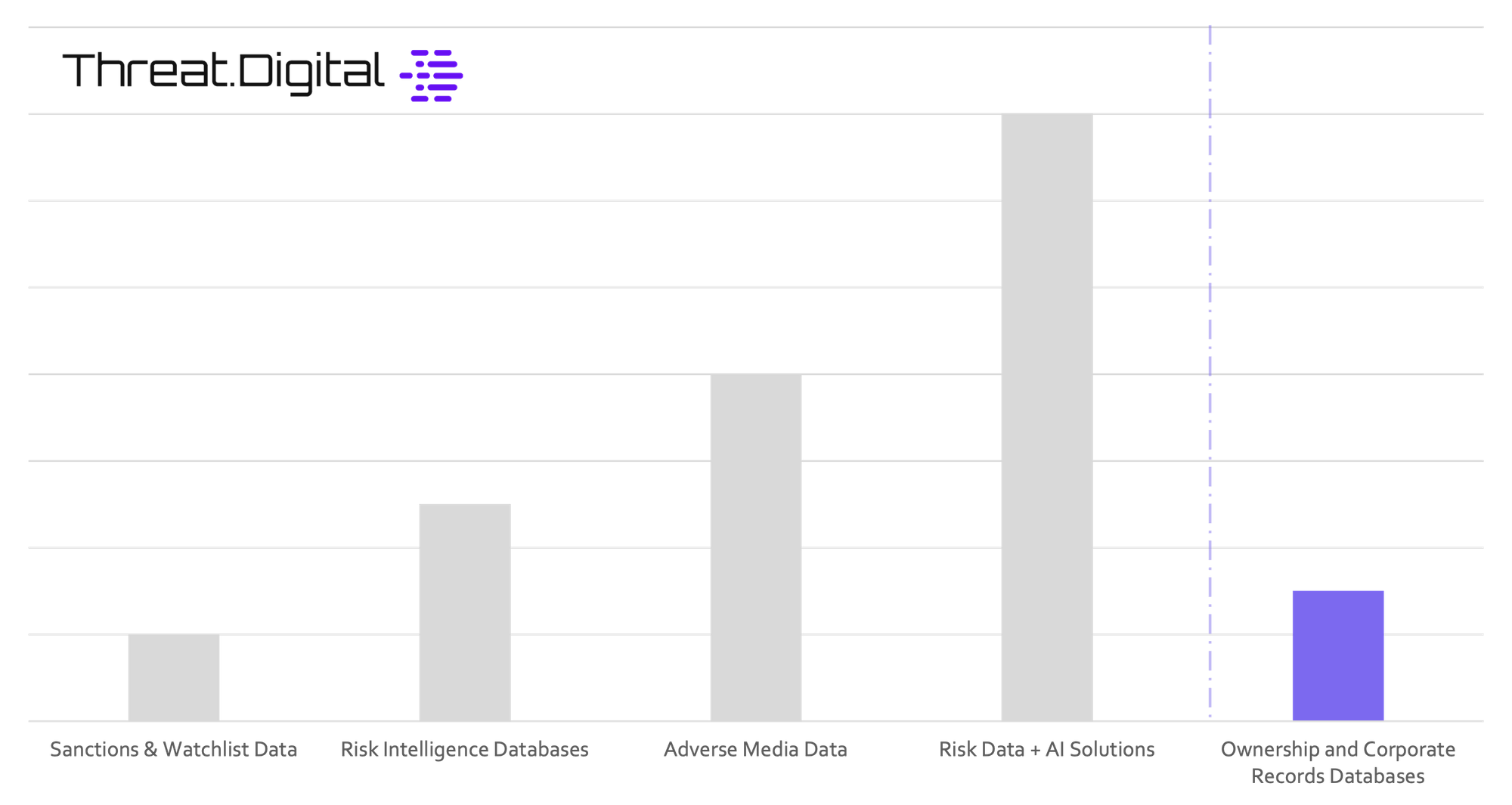
Risk Data Overlap
It is important to understand the above solutions in isolation, but even more interesting is to see how the types of information provided in each category overlap with the other groups. For example, all sanction data would also be included in a Risk Intelligence Database solution, and while the data in the company ownership category relevant to risk is limited and highly specific, much of the information in that category won't be found in the other datasets unless specifically integrated, and while a pure adverse media solution may cover most of the information included in a Risk Intelligence Database, it's lack of structure and curation make it unsuitable for high volume screening use case.
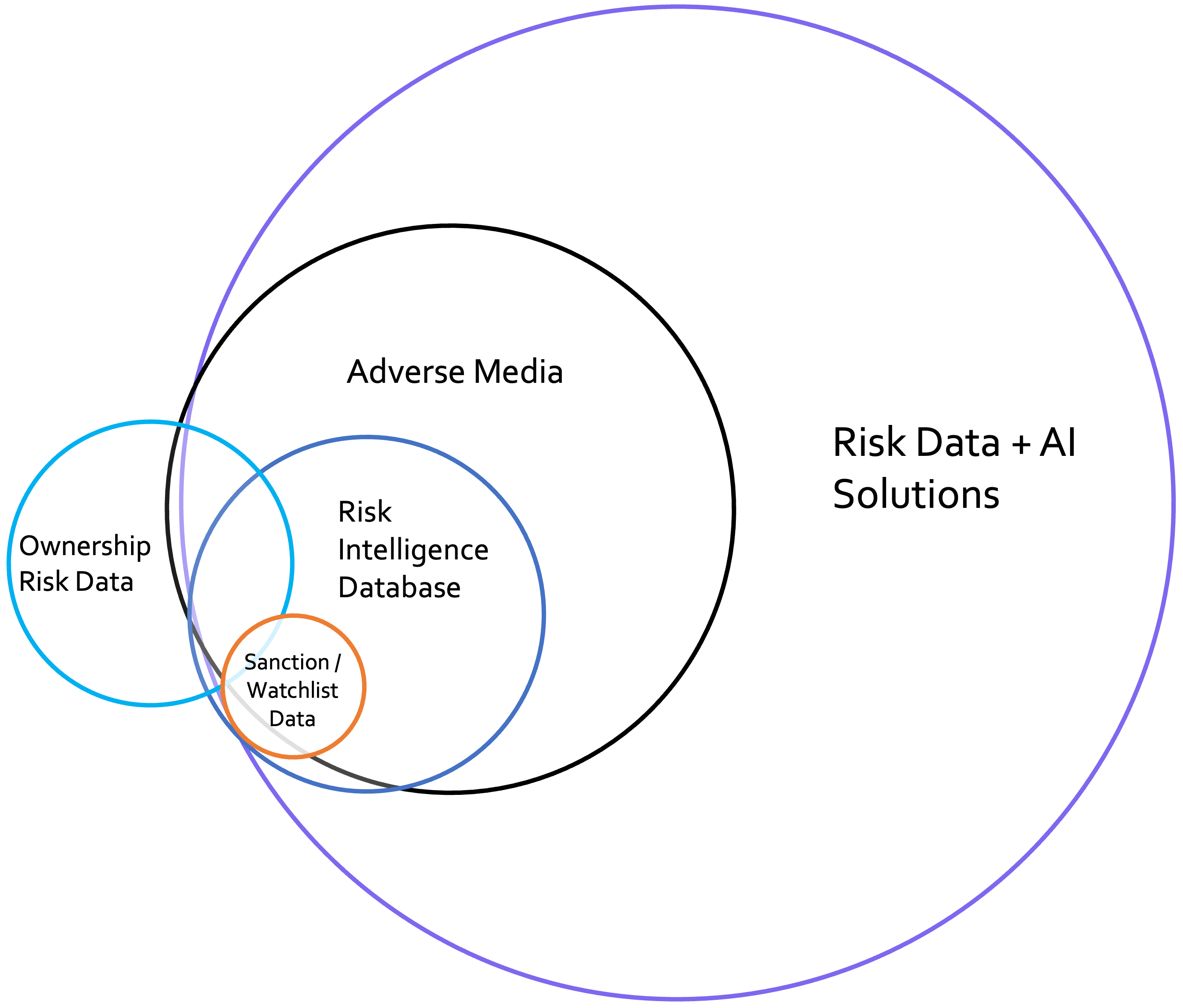
In Closing
Understanding the nuances of each category empowers compliance professionals to adopt a nuanced approach, leveraging appropriate tools to meet regulatory requirements effectively while mitigating financial crime risks. Whether it's basic sanction screenings or cutting-edge AI-driven solutions, the key lies in aligning risk intelligence data with the organization's specific needs and risk appetite.
Learn more about DiligenAI from Threat.Digital!