The False Positive Race is On!
Understanding false positives in name screening. A real-world perspective from Christian Focacci, CEO of Threat.Digital.
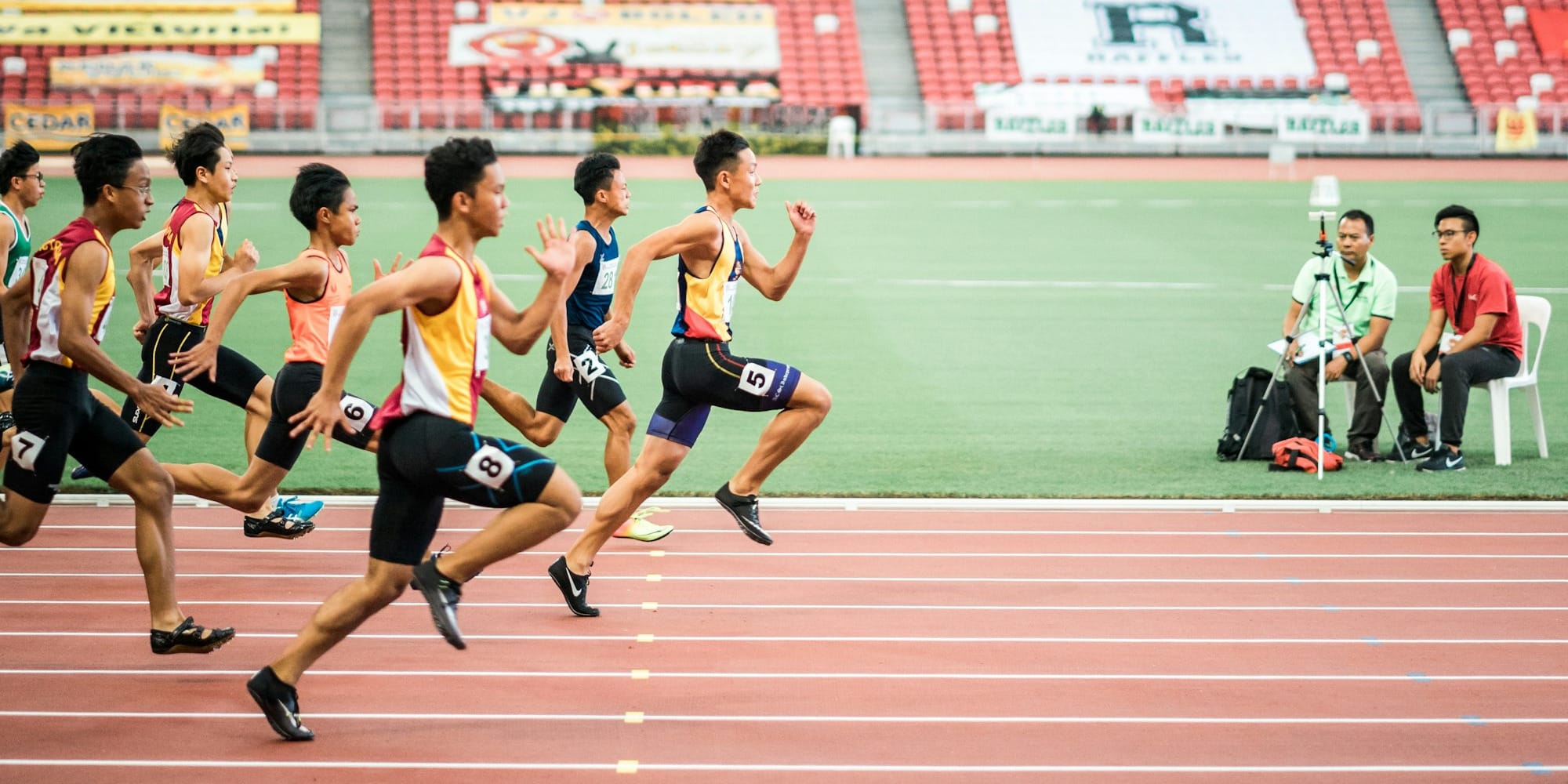
I’ve recently noticed a wave of posts on LinkedIn from vendors talking about false positives in name screening (sanctions, watchlist, adverse media, etc.). It’s a familiar theme, and one that has been a main pain point in financial crime compliance since the first name was screened against a list. What’s different now is the technology. For the first time in a long while, we’re seeing tools that have the potential to actually shift how this problem is handled, specifically the adoption of large language models ('LLMs' - which I have just settled to call AI).
I’ve been working in financial crime compliance for nearly 20 years, starting out in large financial institutions where I spent way too much time reviewing and clearing screening alerts. Over the past decade, I’ve focused on building the technology behind these systems, including platforms used by major global institutions, including one that screens over 20 million names per day. Within that time, I haven’t seen anything as impactful in this space as the application of large language models. Other than the rise of the internet, which revolutionized how information is accessed, this is the first major leap in technology that has the potential to greatly change how compliance work is conducted.
So for this post, I wanted to take a step back and walk through the entire issue: why false positives happen, how to reduce them, and what’s actually working in the field today. I’ll cover rule-based filtering, tuning data sources, and of course, the application of AI. I also highlight a few examples I’ve seen across the industry. My own company works in this space too, and I’m probably a little biased, but I’ve been around long enough to know that the real competition for compliance technology startup isn't usually another vendor. It's the status quo.
My goal here is to share a straightforward, experience-based perspective on one of the biggest challenges in screening. Whether you're hands-on with alerts or exploring smarter tools for your team, I hope this helps you make sense of what's out there and where things are heading.
What Causes False Positives?
Most false positives happen when a screening system flags a name that sounds or looks similar to someone on a sanction or watchlist, but isn’t actually the same person or entity. Here’s why that happens:
- Many names are common.
- Spelling variations and transliterations are frequent.
- Screening tools often err on the side of caution, using broad matching techniques to avoid missing anything important.
The result? You end up with long queues of alerts, most of which turn out to be irrelevant. The kicker? This is actually by design to ensure that nothing is missed.
So How Do We Limit False Positive Matches?
Fortunately, there are several practical ways to reduce false positives without compromising accuracy. Below are the top three key strategies that organizations can use to clean up their alert queues and boost efficiency.
1. Use Rules to Filter by Contextual Identifiers
One of the simplest and most effective ways to reduce false positives is by comparing identifiers that provide more context about the person or entity being screened. This might include details like:
- Date of birth
- Country or region
- Nationality
- Gender
- Occupation
When screening results include this kind of information, organizations can apply rules to filter out unlikely matches. For example, if a sanctioned individual is listed as 72 years old and residing in another country, that match can be excluded if the person being screened is a 35-year-old local resident.
The limitation here is data availability. Many sanction lists and risk databases do not consistently include these identifiers. On the flip side, the internal data used for screening may also lack these fields. If either side of the match is missing key details, this method becomes less effective. Still, it remains the lowest-hanging fruit and is usually the first place companies should start.
2. Tune and Tailor Your Data Sources
Another effective way to reduce noise is to take a closer look at the data sources themselves. Not all lists are equally relevant to every organization.
For example, if your business operates only in Australia and screens customers with no ties to Latin America, it might not make sense to include a tax blacklist from Brazil in your screening data. Including irrelevant sources can generate a disproportionate number of alerts with very little actual risk.
Most data providers also allow you to filter lists based on certain criteria. For politically exposed persons (PEPs), for instance, you may be able to limit screening to higher-risk levels, such as heads of state or individuals from high-risk jurisdictions. Reducing the scope of your sources in a thoughtful way can eliminate a significant portion of irrelevant alerts.
It is important to note that this must be done carefully. Removing sources can introduce risk if not well documented and justified. However, when guided by business logic and risk tolerance, tuning your data inputs can be a highly effective lever.
3. AI - Use Large Language Models to Add Contextual Review
The most significant advancement in reducing false positives has come from the application of large language models (LLMs) for name screening. These models are capable of reading and understanding context in ways traditional systems cannot.
LLMs do not just look at whether two names are similar. They can evaluate the content surrounding the name: the role, location, biography, and other contextual clues to determine whether a match is likely to be valid. This kind of review can act as a second layer after traditional fuzzy matching, dramatically reducing the number of false alerts that reach compliance teams.
To better understand the impact, we recently ran a benchmark test comparing two approaches:
- Traditional fuzzy matching only
- Fuzzy matching followed by LLM review from our DiligenAI system
Here’s how the two methods performed:
Method | False Positive Reduction | Precision | Recall | F1 Score |
---|---|---|---|---|
Fuzzy Matching Only | Baseline | ~7% | 100% | ~13% |
Fuzzy + LLM Review | Over 94% reduction | ~63% | 100% | ~77% |
Both approaches identified all true matches, but the LLM-enhanced method delivered much higher precision. This brought the F1 score, a measure of overall effectiveness, up almost sixfold.
The result is a system that’s dramatically more efficient. The LLM-enhanced approach reduced false positives by over 94 percent, while maintaining the same recall*. In practical terms, this means fewer unnecessary alerts, less manual work, and better use of your team’s time.
The downside of this approach is that (at this point) frontier AI models are needed to reach these levels, and there is a much higher cost per name to screen. That would be offset with the time savings from having an analyst look at those lower quality alerts, but it is still worth understanding.
*The fuzzy name matching threshold was tuned so that it would have a recall of 100% against a benchmark list of different name matching scenarios.
Finding the Right Balance
Every screening program has to balance risk sensitivity with operational efficiency. Too many false positives slow everything down. Too few alerts increase the chance of missing something critical.
The best approach is to use the available false positive reduction approach in coordination. Rule-based filtering, intelligent data tuning, and AI-driven review each contribute to better performance and faster workflows.
LLM-based screening is still emerging, but we’ve been using this technology in production for over two years, and the precision gains are already significant.
If you are interested in learning more about how applied LLMs and AI can help your organization, we are happy to share what we’ve learned. The technology is evolving quickly, and now is a great time to explore its potential.